1. Department of Crop Science, Makerere University, P .O. Box 7062, Kampala, Uganda
2. Kenya Sugar Research Foundation, P.O.Box 44-40100, Kisumu, Kenya
3. Maseno University Private bag, Maseno, Kenya
4. Biosciences eastern and central Africa (BeCA) ILRI hub, P.O.Box 30709-00100, Nairobi, Kenya
5. Kenya Industrial Research and Development Institute, P.O.Box 30650-00100, Nairobi, Kenya
6. Ministry of Agriculture and Livestock, Chibombo District. P.O.Box 94, Zambia

Author

Correspondence author
Molecular Plant Breeding, 2014, Vol. 5, No. 6 doi: 10.5376/mpb.2014.05.0006
Received: 23 Apr., 2014 Accepted: 11 May, 2014 Published: 30 May, 2014
Genetic distance; Genetic diversity; Simple sequence repeats (SSR) markers; Sweet sorghum
Interest in exploiting sweet sorghum (Sorghum bicolor (L.) Moench) as a biofuel crop is growing due to its rich stalk sugar content (Wang et al., 2009). Many breeding programs are working towards development of high-yielding varieties and hybrids with higher sugar content, resistance to diseases, drought tolerance and good agronomic traits (Klein et al., 2008). Significant breakthrough has been made in developing and releasing sorghum hybrids and varieties for commercial cultivation both in India and elsewhere (Kumar et al., 2011). Genetic similarity estimates among genotypes are important in selecting parental combinations for creating segregating populations to maintain genetic diversity in a breeding programs (Becelaere et al., 2005), develop mapping populations for detecting quantitative trait loci (QTL) (Varshney, 2011) and categorize lines into heterotic groups for hybrid crop breeding (Menz et al.,2004). Simple sequence repeats (SSR), also known as microsatellites, are based on tandem repeats of one to six core nucleotide elements. Different studies have recommended the use of SSR markers in analyses of genetic diversity due to their high degree of polymorphism (Geleta et al., 2006; Ali et al., 2008; Shehzad et al., 2009). Simple sequence repeats are co dominant markers dispersed throughout the genome, and have multiple alleles that often have conserved loci between related species (Brown et al., 1996; Schulman, 2006). The SSRs are able to discriminate among closely related individuals, and have advantage over other markers in their ability to trace pedigrees in plants (Powell et al., 1996). In sorghum, several studies have been conducted involving SSR markers either alone or in combination with other marker types (Casa et al., 2005; Ali et al., 2008; Klein et al., 2008; Murray et al., 2009). In sweet sorghum, genetic diversity has been successfully determined using amplified fragment length polymorphism (AFLP) and simple sequence repeat (SSR) markers. Due to their high values for polymorphic information content (PIC) and the Shannon diversity index (Geleta et al., 2006), these marker types were adequate for use in these species. Sweet sorghum gene pool creation has not received much attention mainly because it was not considered to be among important crops in Kenya, and the pedigree information is scarce and incomplete. The study was therefore undertaken to assay genetic diversity among a collection of sweet sorghum genotypes using SSR markers to identify specific genotypes exhibiting highest levels of polymorphism i.e. genotypes bearing a maximum of favourable alleles with the aim of improving the genetic base of the current cultivated sweet sorghum to support a breeding programme that will provide Kenyan and African farmers with high-yielding sweet sorghum varieties.
Results
Marker characterization and allele frequencies
The allele sizes among the genotypes for the 11 microsatellites varied from 98 to 244 bp (Table 1). Number of repeats in the SSR motif had strong correlation with allele number and their polymorphism information content (Table 2). The 11 SSRs revealed a total of 86 alleles with a mean of 8 alleles per marker (Table 2). The allele number ranged from 3 (mSbCIR 246) to 19 (Xtxp 012). All the markers were polymorphic (Table 2). The PIC value over the 11 SSR markers averaged 0.53, ranging from 0.09 for marker Xcup 63 to 0.89 for marker Xtxp 012. The mean level of heterozygosity per SSR marker was 0.17 (Table 2). Heterozygosity level ranged from 0.00 for marker Xtxp 145 to 0.84 for marker mSbCIR 246. Marker Xtxp 012 had the highest gene diversity of 0.9 while marker Xcup 63 had the lowest gene diversity of 0.09. The mean gene diversity per SSR marker was 0.56 (Table 2). All the loci were polymorphic with Zambian population having the highest number of private alleles (Table 3).
.png)
Table 1 The 11 SSR markers used in this study, the dyes used to label them, repeat motif, chromosome number and allele size range
|
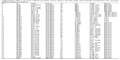
Supplementary Table 1 86 sweet sorghum genotypes used in genetic diversity study, their genotype identification (GI), genotype name and region of collection
|
.png)
Table 2 Summary of allele frequency, allele number and diversity indices of 86 sweet sorghum genotypes
|
.png)
Table 3 Table of genetic diversity for each sweet sorghum populations analyzed in this study
|
Population structure
There was a clear genetic differentiation among individuals within populations, and within the individuals using significance tests based on 1,000 permutations (Table 4). The variability among the populations was low as 3 % but amounted to 22% and 75 % within individual genotypes and among individuals respectively. The FST value was 0.034.
.png)
Table 4 AMOVA partitioning SSR variation, among populations, among individuals within populations, and within individuals in 86 sweet sorghum genotypes
|
Genetic diversity within regions
The allele frequency based pair-wise genetic distances between the countries calculated using Power- Marker version 3.25 revealed the relatedness of genotypes on a country by country basis (Table 5). Genotypes from Kenya and Brazil were the most distant at 0.303 whereas genotypes from Kenya and Zambia were the closest at 0.070.
.png)
Table 5 Genetic distance matrices between countries calculated according to Nei (1987) for the 86 sweet sorghum genotypes
|
Cluster analysis
The pair-wise dissimilarity indices among the sweet sorghum genotypes were estimated using allelic data by simple allele matching followed by cluster analysis using unweighted neighbor-joining algorithm. All the 86 genotypes fell into two (I and II) clusters corresponding mainly to their geographic origin and pedigree. The biggest cluster, cluster I had 83 genotypes with cluster II having 3 genotypes (Z35, Z42 and K86), Figure 1.
.png)
Figure 1 Dendogram (radial axis) of 86 sweet sorghum genotypes revealed by cluster analysis of genetic similarity estimates generated by Nei coefficient based on 11 SSR markers
|
Discussion
With the availability of complete sorghum genomic sequence (Paterson et al., 2009) simple sequence repeats (SSRs) have become the preferred markers of choice for studying genetic diversity of sorghum owing to their co-dominance, multi-allelic nature, ease of use and repeatability. Eight alleles per marker shown in Table 2 was higher than the average of 5.9 previously reported in elite sorghum lines (Smith et al., 2000) but lower than the average reported in the inbreds of sorghum (Menz et al. 2004) who reported 8.7 alleles per locus. The average number of alleles revealed per SSR locus detected was higher to that detected by Schloss et al. (2002), Thudi and Fakrudin, 2011). This could be due to levels of polymorphism of SSR markers, the diversity of genotypes and the sensitivity of DNA fragment separation systems. Mean level of heterozygosity per SSR marker of 0.17 in table 2 is similar to what was reported by Ngugi and Moraa (2012). PIC provides an estimate of the discriminatory power of a locus or loci by the number of alleles expressed and the relative frequencies of those alleles. According to PIC values, 2 markers (Xcup 53 and Xcup 63) were slightly informative (PIC<0.25 with mean=0.095), 2 markers (Xtxp 273 and Xtxp 145) were reasonably informative (0.25<PIC<0.5, mean PIC=0.42), 5 markers (mSbCIR 246, Xcup 14, Xcup 12, mSbCIR 283 and mSbCIR 329) were highly informative (0.5<PIC>0.75, mean PIC=0.64). The SSR Xcup 63 has been identified as rare in an earlier study (Ali et al., 2008). The FST value of 0.034 in Table 4 indicates negligible genetic differentiation among the population analyzed. FST values up to 0.05 indicate negligible genetic differentiation whereas >0.25 means very great genetic differentiation within the population analyzed. This demonstrates that sweet sorghum cultivars from Argentina, Brazil, Kenya, United States of America and Zambia, are very closely related. Efforts to widen genetic variability and improve sweet sorghum through exchange of cultivars for breeding within these countries are unlikely to yield useful results. Similar observation had also been made by Geleta et al. (2006)
Marker series Xtxp 012, Xtxp 021 which were of genomic origin were highly polymorphic compared to gene-based marker series Xcup 53 and Xcup 53 (Table 2). Genic SSRs have been reported to be less polymorphic compared with genomic SSRs in crop plants because of greater DNA sequence conservation in transcribed regions (Schloss et al., 2002).
SSRs with di-nucleotide repeats are the most polymorphic marker class followed by tri-, tetra- and penta-repeat units. A direct relationship exists between marker information content and the number of repeat units (Weber 1990; Innan et al., 1997; Schloss et al., 2002). The observed inbreeding coefficient (FIS=0.77) was high probably as a consequence of self fertitilization. Ellstrand and Foster (1983) reported similar level of selfing. The gene diversity observed in this study (Mean PIC=0.53) is closer to the diversity value (0.40, 0.46, 0.62, 0.58) reported by Ali et al. (2008), Schloss et al. (2002), Agrama and Tuinstra (2003) and Smith et al. (2000), respectively. The SSR loci mSbCIR 329, Xcup 14 and Xcup 12 were rich in allelic diversity exhibiting (9-19) alleles with highest PIC of 0.89. Thus, these primers could be of great use in DNA fingerprinting to characterize sweet sorghum genetic stocks in view of the emerging needs for Distinctiveness, Uniformity and Stability (DUS) characterization and plant varietal registration.
Conclusion
Pairs of genotypes, which can be exploited to select parents for hybrid development to maximize the sugar content and total biomass and for development of segregating populations to map genes controlling sugar content in sweet sorghum, were identified. The results presented here demonstrate that sweet sorghum cultivars from Argentina, Brazil, Kenya, United States of America and Zambia, are very closely related. Efforts to widen genetic variability and improve sweet sorghum through exchange of cultivars for breeding within these countries are unlikely to yield useful results. Similar observation had also been made by Geleta et al. (2006) suggesting improvement of sweet sorghum varieties should access additional new genes. It may therefore be necessary the future breeding strategies are directed at crossing sorghum with some of its close relatives.
Materials and Methods
Germplasm collection
A set of 86 cultivars of sweet sorghum genotypes were selected as follows: Four from Argentina, seven from Brazil, 29 from Kenya (ICRISAT and Moi University), four from United States of America and 42 from Zambia.
DNA extraction
Total genomic DNA was extracted from young leaves (12 days old) of five plants of each line planted in the green house at Biosciences eastern and central Africa (BeCA) ILRI hub in Nairobi, Kenya. Five (5) plants per genotype were harvested and pooled into microtubes tubes. DNA was extracted from leave samples using a modified CTAB protocol (Mace et al. 2003). Two steel beads were put in each well of strip tubes (Greentree Scientific, USA) that were processed in a Geno Grinder 2000. The samples were placed in microtubes and then, 450 μL preheated (65ºC) Extraction Buffer (EB) (3% (w/v) CTAB, 1.4 M NaCl, 0.2% (v/v) β-Mercapto-ethanol and 20 mM EDTA) was added and ground using the Geno-grinder. The quantity and quality of the DNA were checked using a Nano-drop spectrophotometer.
PCR and SSR assay
Eleven SSR primers from a reference microsatellite kit used to assess genetic diversity of Sorghum bicolor (Billot et al., 2012) were selected based on their clear polymorphic patterns and on their position in the sorghum genome, covering ten linkage groups or chromosomes. Upon dilution of DNA samples to 20 ng/μL, a 10 μL PCR mix consisting of 20 ng of DNA, 10× reaction buffer, 2 mM MgCl2, 0.2 mM dNTPs, 2 pmols of forward and reverse primers, 0.5 U Taq polymerase was prepared for each genotype. Temperature cycling was carried out using the GeneAmp PCR systems 9700 (PE-Applied Biosystems), using a program with an initial denaturation at 94°C for 60 s followed by 35 cycles at 94°C for 60 s, 55°C for 60 s, and 72°C for 60 s with a final hold at 72°C for 20 min. After the PCR, a few accessions in each primer were randomly selected and their PCR products (3 uL) run on agarose (2%) gel electrophoresis stained with gel red (2.5 uL) at a voltage of 100 V for 30 minutes. Genotyping was carried out by capillary electrophoresis using the ABI PRISM 3730 (Applied Biosystems). DNA fragments were denatured and size-fractioned using capillary electrophoresis. The SSRs used in the study represented di-, tri-, tetra- and penta- nucleotide repeat units. The peaks were sized and the alleles identified using Gene-Mapper software and the internal GS500 (-250) LIZ size standard.
Cluster analyses
Dissimilarity indices were estimated using allelic data by simple allele matching and cluster analysis based on unweighted neighbor-joining (Gascuel, 1997) were carried using DARwin 5.0 dissimilarity analysis software (Perrier et al., 2003). To ascertain the statistical strength of genetic relationships identified through this analysis, bootstrapping of the data (10,000 permutations) was performed. The total number of alleles detected, the number of common alleles with allelic frequencies of at least 5%, the observed size range (in base pairs; bp), the allele size differences (in bp), the polymorphism information content (PIC) values (Smith et al., 2000), and frequencies of unique alleles were calculated for each SSR marker using PowerMarker Version 3.25 (Liu and Muse, 2005).
Data analysis
Alleles were called and identified using Gene-Mapper version 3.7. Data was subjected to Allelobin software to check the quality of the SSR markers. Data generated from Allelobin was analyzed using Power-Marker version 3.25 to calculate the Polymorphic Information Content (PIC), heterozygosity, number of alleles for each marker, percentage of polymorphic loci estimates, and genetic diversity among the genotypes and their genetic distances. Allele and genotype frequencies were scored using haplotype diversity values with PowerMarker version 3.25 (Schenider et al., 2000). Darwin Version 5.0 software was used to calculate the principle coordinate analysis (PoCA) and clustering among the genotypes. To determine the genetic relationships and differentiation; the 86 sweet sorghum accessions were clustered based on the matrix of genetic similarities using the Un-weighted Pair Group Method using the Arithmetic Averages (UPGMA) algorithm. Dissimilarity Index was calculated from allelic data by simple matching. The distances were computed for microsatellite data (11 loci) and trees constructed using the neighbour-joining method with DARwin Version 5.0 software. The genetic distance between genotypes was subjected to sequential agglomerative hierarchical nested (SAHN) with un-weighted, pair-group analysis (UPGMA) using Dice’s indices as provided in DARwin 5.0. Major clusters were generated from Nei (1987) genetic distance matrices. Analysis of molecular variance (AMOVA) (Excoffier et al., 1992) was used to partition SSR variation among groups. Significance levels for variance component estimates were computed by a non-parametric permutation procedure using 100 permutations. AMOVA and Fst indices were calculated using the GenAlEx program, version 6.5 (Peakall and Smouse, 2012).
Acknowledgment
This research was supported by Africa Bioscience Challenge Fund (ABCF) and Kenya Sugar Research Foundation (KESREF). We gratefully acknowledge the financial support provided to the Biosciences eastern and central Africa Hub at the International Livestock Research Institute (BecA-ILRI Hub) by the Australian Agency for International Development (AusAID) through a partnership between Australia's Commonwealth Scientific and Industrial Research Organization (CSIRO) and the BecA-ILRI Hub; and by the Syngenta Foundation for Sustainable Agriculture (SFSA); the Bill & Melinda Gates Foundation (BMGF); Swedish Ministry of Foreign Affairs through the Swedish International Development Agency (Sida), which made this work possible.
We extend special thanks to Dr. Rob Skilton from Biosciences eastern and central Africa (BeCA) ILRI-Hub for technical and administrative leadership. Dr.Chrispine Omondi, Director, Kenya Sugar Research Foundation for technical guidance, ICRISAT-Kenya, Embrapa-Brazil, Zambia Agriculture Research Institute-Sorghum Breeding Program, Professor S.Gudu of Moi University and Mr. Peter Kegode of Mark Consulting Services Limited for providing the seeds of sweet sorghum cultivars used in this study.
We gratefully acknowledge the excellent technical support provided by SegoliP, the BecA-ILRI Hub's sequencing and genotyping research support unit.
Agrama H.A., and Tuinstra M.R., 2003, Phylogenetic diversity and relationships among sorghum accessions using SSRs and RAPDs, Afr. J. Biotechnol., 2: 334-340
Ali M.L., Rajewski J.F., Baenziger P.S., Gill K.S., Eskridge K.M., and Dweikat I., 2008, Assessment of genetic diversity and relationship among a collection of US sweet sorghum germplasm by SSR markers, Molecular Breeding, 21: 497‑509
http://dx.doi.org/10.1007/s11032-007-9149-z
Brown S.M., Hopkins M.S., Mitchell S.E., Senior M.L., Wang T.Y., Duncan R.R., Gonzalez-Candelas F., and Kresovich S., 1996, Multiple methods for the identification of polymorphic simple sequence repeats (SSRs) in sorghum [Sorghum bicolor (L.) Moench.], Theor. Appl. Genet., 93: 190-198
http://dx.doi.org/10.1007/BF00225745
Casa A.M., Mitchell S.E., Hamblin M.T., Sun, H., Bowers J.E., Paterson A.H., Aquadro C.F., and Kresovich S., 2005, Diversity and selection in sorghum: simultaneous analyses using simple sequence repeats, Theoretical and Applied Genetics, 111: 23‑30
http://dx.doi.org/10.1007/s00122-005-1952-5
Claire Billot, Ronan Rivallan, Mbaye Ndoye Sall, Daniel Fonceka, Monique Deu, Jean-Christophe Glaszmann,Jean-Louis Noyer, Jean-François Rami,Ange-Marie Risterucci, Patrick Wincker, Puna Ramu, and Charles T. Hash, 2012, A reference microsatellite kit to assess for genetic diversity of sorghum bicolor (poaceae). American Journal of Botany: 10.3732/ajb.1100548
http://dx.doi.org/10.3732/ajb.1100548
Doyle J.J., and Doyle J.L. 1990., Isolation of plant DNA from fresh tissue, Focus, 12: 13‑15
Ellstrand N.C., Foster K.W., 1983, Impact of population structure on the apparent out crossing rate of grain sorghum (Sorghum bicolor), Theor. Appl. Genet., 66: 323-327
Excoffier L., Smouse P.E., and Quattro J.M., 1992, Analysis of molecular variance inferred from metric distances among DNA haplotypes: Application to human mitochondrial DNA data, Genetics, 131: 479-491
Gascuel O., 1997, Concerning the NJ algorithm and its unweighted version, UNJ. In: Mathematical Hierarchies and Biology. DIMACS workshop, Series in Discrete Mathematics and Theoretical Computer Science, Am. Mathematical Soc., 37: 149-170
Geleta N., Labuschagne M.T., and Viljoen C.D., 2006, Genetic diversity analysis in sorghum germplasm as estimated by AFLP, SSR and morpho‑agronomical markers, Biodiversity and Conservation, 15: 3251‑3265
http://dx.doi.org/10.1007/s10531-005-0313-7
Innan H., Terauchi R., and Miyashita T., 1997, Microsatellite polymorphism in natural populations of the wild plant Arabidopsis thaliana, Genetics, 146: 1441-1452
Klein R.R., Mullet J.E., Jordan D.R., Miller F.R., Rooney W.L., Menz M.A., Franks C.D., Klein P.E., 2008, The effect of tropical sorghum conversion and inbred development on genome diversity as revealed by high-resolution genotyping, Plant Genome, 1: 12-26
Kumar A.A., Reddy B.V.S., Sharma H.C., Hash C.T., Srinivasa Rao P., Ramaiah B., Sanjana Reddy P., 2011, Recent advances in sorghum genetic enhancement research at ICRISAT. Am. J. Plant Sci., 2: 589-600
http://dx.doi.org/10.4236/ajps.2011.24070
Mace E.S., Hutokshi K., Buhariwalla H.K., and Crouch J.H., 2003, A high-throughput DNA extraction protocol for tropical molecular breeding programs, Plant Mol. Biol. Rep., 21: 459-46
http://dx.doi.org/10.1007/BF02772596
Menz M.A., Klein R.R., Unruh N.C., Rooney W.L., Klein P.E., and Mullet J.., 2004, Genetic diversity of public inbreds of sorghum determined by mapped AFLP and SSR markers, Crop Sci., 44: 1236-1244
http://dx.doi.org/10.2135/cropsci2004.1236
Nei M., 1987, Molecular Evolutionary Genetics, Columbia University Press, New York
Paterson A.H., Bowers J.E., Bruggmann R., Dubchak I., and Grimwood J., 2009, The Sorghum bicolor genome and the diversification of grasses, Nature, 457: 551-556
http://dx.doi.org/10.1038/nature07723
Peakall R., and Smouse P.E., 2012, GenALEx 6.5: genetic analysis in Excel. Population genetic software for teaching and research-an update.
Powell W.W., Machery G.C., Provan J., 1996, Polymorphism revealed by simple sequence repeats, Trends Gen., 1: 76-83
Shehzad T., Okuizumi H., Kawase M., and Okuno K., 2009, Development of SSR‑ based sorghum (Sorghum bicolor (L.) Moench) diversity research set of germplasm and its evaluation by morphological traits, Genetic Resources and Crop Evolution, 56: 809‑827
http://dx.doi.org/10.1007/s10722-008-9403-1
Schloss S.J., Mitchell S.E., White G.M., Kukatla R., Bowers J.E., Paterson A.H., and Kresovich S., 2002, Characterization of RFLP probe sequences for gene discovery and SSR development in Sorghum bicolor (L.) Moench, Theoretical and Applied Genetics, 105: 912‑920
http://dx.doi.org/10.1007/s00122-002-0991-4
Schneider S., Roessli D., and Excoffier L., 2000, A software for population genetics data analysis, Arlequin ver. 2000 User Manual, Genetics and Biometry Lab, Dept. of Anthropology, University of Geneva, Geneva
Smith C.W., Frederiksen R.A., 2000, Sorghum: origin, history, technology and production. Wiley, New York. Preface, pp vii–ix
Varshney R.K., 2011, Application of next generation sequencing and genotyping technologies to develop large-scale genomic resources in SAT legume crops. In: Muralidharan K and Siddiq EA (eds.). Genomics and crop improvement: Relevance and Reservations. Institute of Biotechonology, Acharya NG Ranga Agricultural University, Rajendranagar, Hyderabad 500030, India
Wang M.L., Zhu C., Barkley N.A., Chen Z., Erpelding J.E., Murray S.C., Tuinstra M.R., Tesso T.R., Pederson G.A., Yu J., 2009, Genetic diversity and population structure analysis of accessions in the US historic sweet sorghum collection, Theor. Appl. Gen., 120: 13-23
http://dx.doi.org/10.1007/s00122-009-1155-6